Data wrangling skills are so integral to the job, many leading tech companies typically ask new data science candidates to perform a series of data transformations, including merging, ordering.
- Data Wrangling In R Cheat Sheet 2019
- Data Wrangling In R Cheat Sheet Excel
- Data Wrangling In R Cheat Sheet Pdf
- Tidyverse Cheat Sheet Pdf
- Retain only rows with matches. Fulljoin(x, y, by = NULL, copy=FALSE, suffix=c(“.x”,“.y”),) Join data. Retain all values, all rows. Use by = c('col1', 'col2', ) to specify one or more common columns to match on. Le'join(x, y, by = 'A') Use a named vector, by = c('col1' = 'col2'), to match on columns that.
- Data Wrangling with dplyr and tidyr Cheat Sheet Tidy Data - A foundation for wrangling in R F MA F MA & In a tidy data set: Each variable is saved in its own column Syntax - Helpful conventions for wrangling. Report Data Wrangling Cheat Sheet - RStudio.
- This cheat sheet is inspired by the data wrangling cheat sheets from RStudio and pandas. Examples are based on the Kaggle Titanic data set. Created by Tom Kwong, November 2020. V0.22 rev1 Page 2 / 2 gdf = groupby(df,:pclass) gdf = groupby(df, :pclass,:sex) Group data frame by one or more columns.
10.1 Scoped verbs vs. purrr
It can be easy to get confused between purrr and scoped verbs. The following diagram illustrates which to use for different combinations of inputs and outputs. For example, use a scoped verb if you want to start and end with a tibble, but purrr if you want to start with a tibble and end up with a vector.
10.2 Suffixes
suffix | use when |
---|---|
_all | you want to apply the verb to all columns |
_at | you want to apply the verb to specified columns |
_if | you want to apply the verb to all the columns with some property |
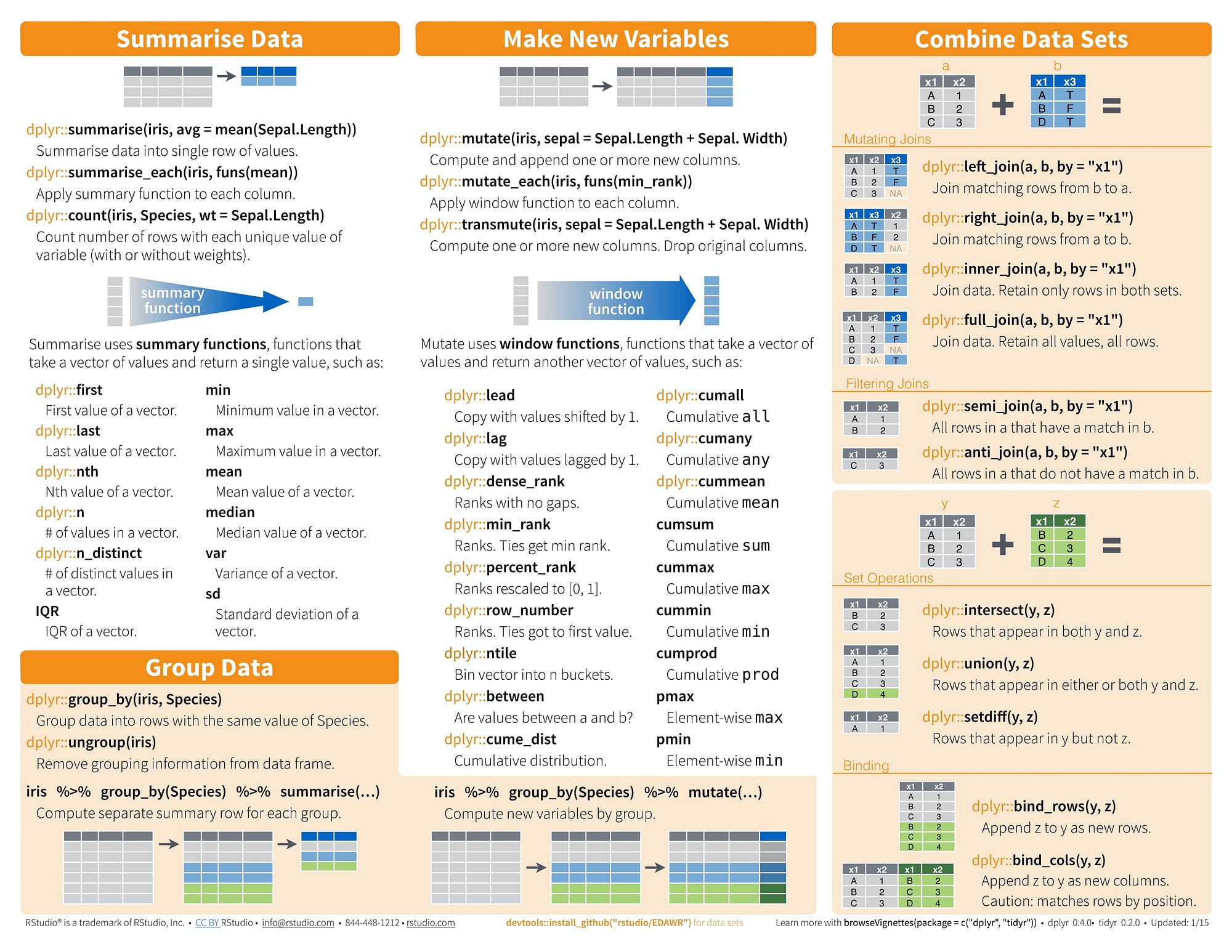
10.3 Examples
10.3.1mutate()
, summarize()
, select()
, and rename()
10.3.1.1 Named functions
Verb | Example | Example explanation |
---|---|---|
summarize_all | summarize_all(mean) | finds the mean of all variables |
summarize_at | summarize_at(vars(x, y), mean) | finds the mean of variables x and y |
summarize_if | summarize_if(is.double, mean) | finds the mean of all double variables |
mutate_all | mutate_all(as.character) | converts all variables to characters |
mutate_at | mutate_at(vars(x, y), as.character) | converts variables x and y to characters |
mutate_if | mutate_if(is.factor, as.character) | converts all factor variables to characters |
rename_all | rename_all(str_to_lower) | changes all column names to lowercase |
rename_at | rename_at(vars(X, Y), str_to_lower) | changes the names of columns X and Y to x and y |
rename_if | rename_if(is.double, str_to_lower) | changes the names of double columns to lowercase |
select_all | select_all(str_to_lower) | selects all columns and changs their names to lowercase (better to use rename_all()) |
select_at | select_at(vars(X, Y), str_to_lower) | selects just columns X and Y and changes their names to x and y |
select_if | select_if(is.double, str_to_lower) | selects just double columns and changes their names to lowercase |
10.3.1.2 Extra arguments
verb | example | example_explanation |
---|---|---|
summarize_if | summarize_if(is.double, mean, na.rm = TRUE) | finds the mean, excluding NAs, of all double variables |
summarize_all | summarize_all(mean, trim = 0.1, na.rm = TRUE) | finds the mean of all variables, exluding NAs. Removes the bottom and top 10% of values of each variable before computing mean |
10.3.1.3 Anonymous functions
verb | example | example_explanation |
---|---|---|
summarize_all | summarize_all(~ sum(is.na(.))) | determines the number of NAs in each column |
select_if | select_if(~ n_distinct(.) > 1) | selects only the columns with more than one distinct value |
10.3.2filter()
Data Wrangling In R Cheat Sheet 2019
verb | example | example_explanation |
---|---|---|
filter_all | filter_all(all_vars(!is.na(.)) | finds rows without any NAs |
filter_all | filter_all(any_vars(!is.na(.)) | finds rows with at least one non-NA value |
filter_at | filter_at(vars(x, y), all_vars(!is.na(.)) | finds rows where both x and y are non-NA |
filter_at | filter_at(vars(x, y), any_vars(!is.na(.)) | finds rows where at least one of x and y is non-NA |
filter_if | filter_if(is.double, all_vars(!Is.na(.)) | finds rows where all double variables are non-NA |
filter_if | filter_if(is.double, any_vars(!Is.na(.)) | finds rows where at least one double variable is non-NA |
I reproduce some of the plots from Rstudio’s ggplot2 cheat sheet using Base R graphics. I didn’t try to pretty up these plots, but you should.
I use this dataset
The main functions that I generally use for plotting are
- Plotting Functions
plot
: Makes scatterplots, line plots, among other plots.lines
: Adds lines to an already-made plot.par
: Change plotting options.hist
: Makes a histogram.boxplot
: Makes a boxplot.text
: Adds text to an already-made plot.legend
: Adds a legend to an already-made plot.mosaicplot
: Makes a mosaic plot.barplot
: Makes a bar plot.jitter
: Adds a small value to data (so points don’t overlap on a plot).rug
: Adds a rugplot to an already-made plot.polygon
: Adds a shape to an already-made plot.points
: Adds a scatterplot to an already-made plot.mtext
: Adds text on the edges of an already-made plot.
- Sometimes needed to transform data (or make new data) to make appropriate plots:
table
: Builds frequency and two-way tables.density
: Calculates the density.loess
: Calculates a smooth line.predict
: Predicts new values based on a model.
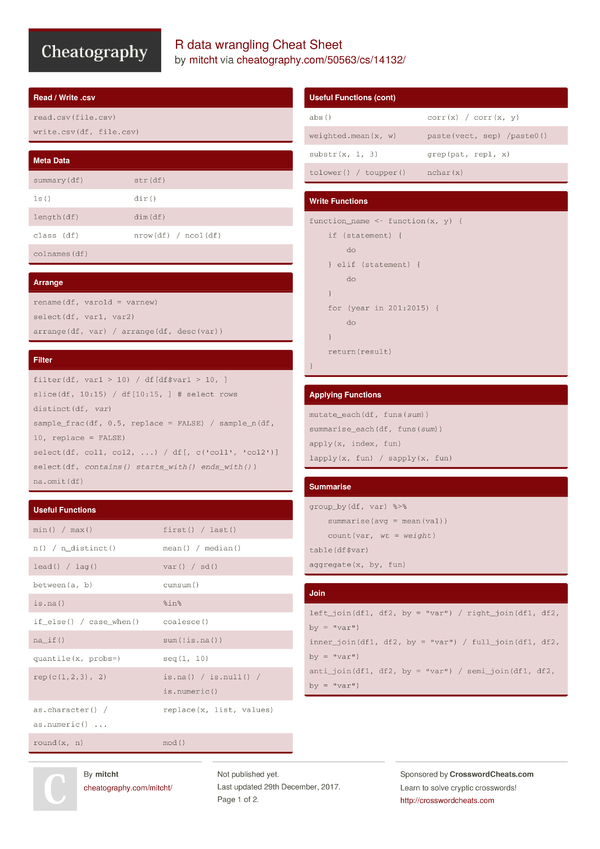
All of the plotting functions have arguments that control the way the plot looks. You should read about these arguments. In particular, read carefully the help page ?plot.default
. Useful ones are:
main
: This controls the title.xlab
,ylab
: These control the x and y axis labels.col
: This will control the color of the lines/points/areas.cex
: This will control the size of points.pch
: The type of point (circle, dot, triangle, etc…)lwd
: Line width.lty
: Line type (solid, dashed, dotted, etc…).
Discrete
Barplot
Different type of bar plot
Continuous X, Continuous Y
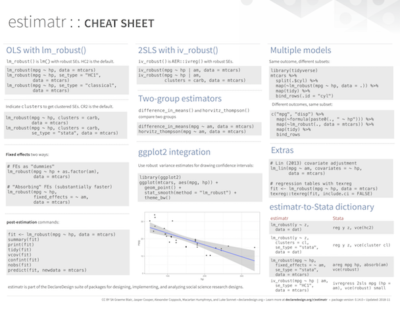
Scatterplot
Jitter points to account for overlaying points.
Add a rug plot
Add a Loess Smoother
Loess smoother with upper and lower 95% confidence bands
Loess smoother with upper and lower 95% confidence bands and that fancy shading from ggplot2
.
Add text to a plot
Discrete X, Discrete Y
Mosaic Plot
Color code a scatterplot by a categorical variable and add a legend.
Data Wrangling In R Cheat Sheet Excel
par
sets the graphics options, where mfrow
is the parameter controling the facets.
Data Wrangling In R Cheat Sheet Pdf
The first line sets the new options and saves the old options in the list old_options
. The last line reinstates the old options.
Tidyverse Cheat Sheet Pdf
This R Markdown site was created with workflowr
